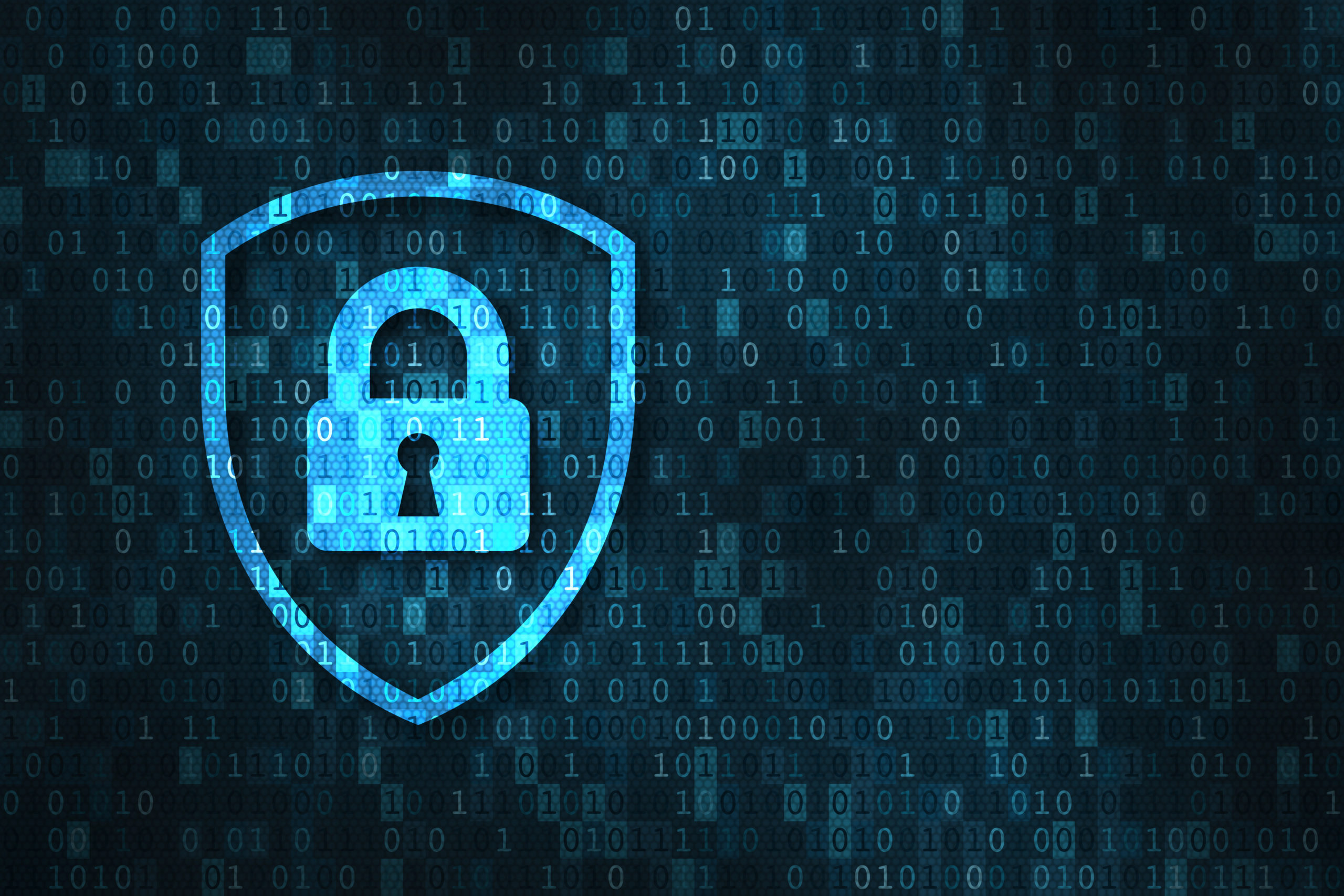
It’s no secret that SOC teams are struggling. The main reason is the sheer volume of data they must collect and analyze to thwart cybercrime. The data sources they need to account for include applications, transactions, IoT devices, mobile devices, and more. And the amount of global information created, replicated, and consumed is projected to increase to more than 180 zettabytes over the next five years, making the data challenge we’re facing even worse.
We can’t expect humans to scale at the same pace as the exponential data growth we’re witnessing. It’s pretty much impossible for that to happen. Therefore, we must augment analysts and threat hunters with automation technologies to rise above this data challenge. Otherwise, security teams won’t stand a chance to defeat cyber adversaries.
Data Modeling = Better Data Insights
To automate effectively, you need good data, and you need to look at it correctly. Most systems look at rows and rows of data in a linear fashion, but it’s incredibly difficult to obtain good insights this way. Instead, you need to look at relationships in the data and model it accordingly.
Dense data, such as endpoint data, is best because it provides a highly granular log of activity from every device across the infrastructure. It can enable systems to create a connected graph of everything happening across the enterprise. When graph-based correlations are made, data is presented in a format that enables AI-powered engines to ask questions and dig deeper to provide analysts and threat hunters with the context they need to understand attackers’ moves.
Spotting Suspicious Activity with Automation
Many of us have used Turbo Tax online. When you go to file, the tax software may ask, “Did you buy a house last year?” If the answer is no, it’s on to the next question. If the answer is yes, it may ask several more questions, such as, “What was the down payment” or, “What was the total cost?” That’s exactly how an AI-powered question engine works when identifying a potential threat.
The attack-tracing AI engine takes an alert, which is very low fidelity, and translates it into a full summary of events by asking a series of questions. In return, the analyst can review pre-investigated and fully contextualized attack traces to mitigate them instead of drowning in data and chasing false positives.
Devo DeepTrace, which was just launched, gives SOC teams this power. Its attack-tracing AI asks potentially hundreds of thousands of questions, quickly performing large quantities of complex alert investigations to autonomously construct traces detailing an attacker’s actions. It then overlays its results against the MITRE ATT&CK framework for additional context and points of reference, reducing investigation times from days to minutes.
Autonomous Threat Hunting
Your security team may be on top of every advisory and alert, but what about the threats they don’t see ? How do you look for what you might be missing?
In traditional threat hunting, humans form a hypothesis and proactively search through networks, endpoints, and datasets looking for potentially malicious activity that was not detected by existing security tooling and alerting. However, because of growing data volumes and an ever-expanding attack surface, there’s too much ground for humans to hunt across on their own. Many SOC teams don’t even have the bandwidth to attempt threat hunting because they lack the capacity and resources.
This is where autonomous threat hunting comes into play — which is one use case of Devo DeepTrace. Similar to traditional threat hunting methods, the threat hunter would formulate a hypothesis as a starting point then leverage automation technologies to search across their infrastructure instead of manually performing the hunt themselves.
For example, a threat hunter could hypothesize that if a Java application is opening a command shell, there could be a potential Log4J issue. A threat hunter could then configure a search based on this hypothesis over a set period of time — hourly, weekly, or monthly — so the system could repeatedly search for it to validate or dispel the theory. If the system were to find something, the AI question engine would kick in to start exploring to understand what has been happening.
The future of threat hunting is here now. And not only will autonomous systems such as DeepTrace help security teams identify threats based on hypotheses they formulate, but they will also help threat hunters formulate new hunts based on what’s been uncovered and learned.
The Future is Autonomous
By implementing a fully autonomous approach to alert triage and threat hunting, security teams stand a fighting chance in the battle against increasingly sophisticated and persistent cybercriminals. Burnout and SOC inefficiency will also reduce, and security teams will become better equipped to focus on activities that will have a greater impact on improving their organization’s overall security posture.
You can learn more information on autonomous threat hunting and the benefits of Devo DeepTrace here.