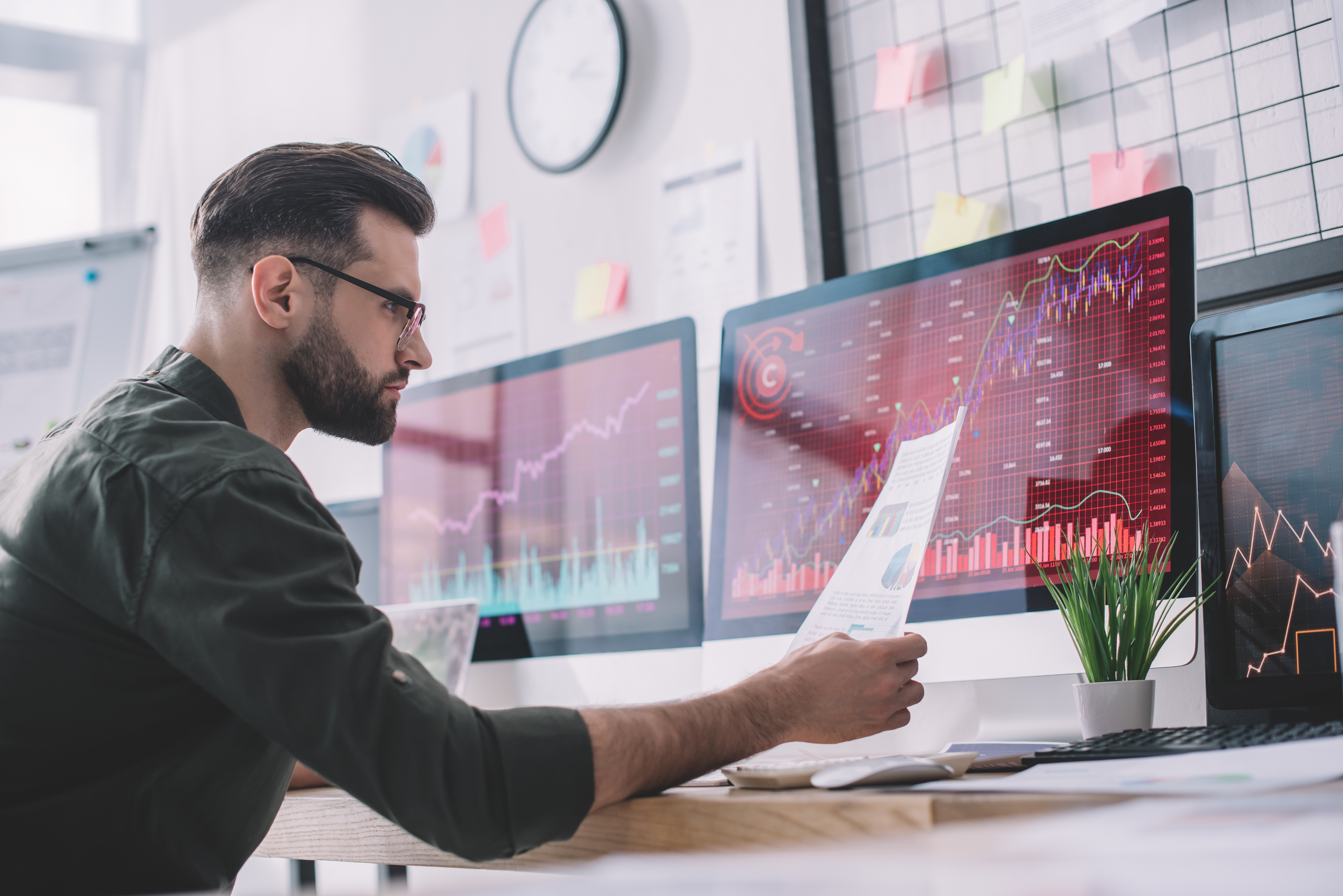
Time sure flies when you have a generative AI chatbot at your side, doesn’t it? While it’s only been one year since ChatGPT was released into the world, the adoption and progress of the technology itself certainly seems impressive. But I wanted to sit down with some AI experts to get their thoughts on the matter. Read on for impressions and insights on Generative AI (GenAI) from Rakesh Nair, Vice President of Engineering and Security Products, and Chaz Lever, Sr. Director of Security Research at Devo.
How have GenAI and ChatGPT progressed over the past year? What security-relevant developments stand out?
Rakesh: GenAI has grown leaps and bounds in the past year. The first extraordinary improvement is the increase in model size–allowing for much better contextual understanding and more pertinent answers. Secondly, GenAI can now take incremental input and fine-tune the model for specific domains, including security, enabling the system to be much more effective in the pragmatic use of this technology.
In the case of security specifically, these specialized models with the vast embedded connections allow us to possibly 1) discriminate between benign and risky behaviors as part of the triage process, 2) generate simplified summaries based on multiple input signals, and finally, 3) make better recommendations for responding to threats based on aggregation of signals generated from event correlation, and anomaly detection.
Chaz: ChatGPT, as a GenAI product offering, has seen numerous improvements and capabilities for the broader public. It’s gained support for understanding more types of content, such as images, audio, and the Internet. Additionally, it incorporated other LLM outputs beyond text (such as images via DALL-E). Over the past year, operational tooling has exploded (plugins, frameworks, etc.).
From a security perspective, LLMs have introduced a whole new attack surface. The natural language interface presents challenges like hallucinations, prompt injection, model inversion, and data poisoning. These are considerable concerns when adopting LLMs in a security setting. As a result, most security implementations have focused on data summarization in, preferably, a non-adversarial environment.
GPT-4 Turbo, the latest version of the technology that powers ChatGPT, supports inputs equal to about 300 pages of a standard book – about 16 times longer than the previous iteration. How does this improvement make adopting AI more viable commercially?
Chaz: Before GPT-4 Turbo, there were other methods for chunking data to analyze datasets that were larger than what was natively supported by LLMs at the time. However, this had several downsides. The user had to set this up manually, and while frameworks and other technologies were built to make this easier, it still wasn’t as simple as passing an entire input to an LLM. This meant the full context of a large input couldn’t all be analyzed by an LLM. The results of chunking large inputs might be suboptimal.
The ability for LLMs to support larger inputs is a solid advancement that makes LLMs easier to work with, and it may make it possible to work with other types of inputs in the future (i.e., ones that can’t be easily chunked).
Rakesh: Gen AI technology will continue to evolve, and performance will continue to improve. The increase in context windows allows us to take on more complex sequences of adversary activity and summarize it or identify more pertinent response actions.
OpenAI recently unveiled a series of AI tool updates, including the ability for developers to create custom versions of ChatGPT called GPTs. Similar to plugins, GPTs can connect to databases, be used in emails, or facilitate e-commerce orders. How do you think this development affects the market, and how will companies start to adopt AI?
Rakesh: This is a potential game-changer, and I expect a cottage industry of companies that would try to create plugins for many applications. The security industry will also see many advancements incorporating this technology into existing products and new applications – especially in the recommendation, search, and training use cases.
Having said that, like any new technology, it will have its teething pains and need some time for the hype to settle down so we can discover more pragmatic use cases as we go through the next few years. The security and privacy implications of giving access to these systems to databases, email, etc need to be further studied.
Chaz: While this change doesn’t represent a fundamental shift in LLM capabilities, it potentially makes developing on top of LLMs easier. This might lead to more experimentation, but it’s unclear how many “useful” developments might stem from this. If you look at the custom GPTs offered by ChatGPT at launch, they’re honestly underwhelming to me.
Nothing suggests how these custom GPTs perform relative to the foundational LLMs they’re built on top of. Beyond branding your own GPT, it’s unclear how much this will advance the state of the art, and it may just result in an explosion of “spammy” GPTs that provide little to marginal benefit.
What, in your opinion, is the Achilles heel of ChatGPT? Where should people and organizations be weary when leaning on this technology?
Rakesh: Gen AI is still, at its core, a large language model that does not understand the semantic meaning of what it suggests. While we can draw inferences, summaries, and recommendations using GenAI from its huge models, it can still be wrong. Newer types of attack vectors are unknown to GenAI models–organizations should ensure they don’t overly rely on this technology.
While the efficacy of generic LLM models are being studied, there is still a considerable amount of effort that needs to go into studying the efficacy of domain-centric models as we specialize. For now, always have a human in the loop.
Chaz: LLMs are the foundational piece of the current GenAI craze and are essentially black boxes. At their foundation, they’re “stochastic parrots”. They’re good at probabilistically guessing the right thing to say, but they do not understand the meaning of what they’re saying. There are questions about using them for fundamentally new things (i.e., they’re not sentient and probably can’t perform tasks outside their training data).
Companies shouldn’t be afraid of this technology but should focus on using it in non-adversarial settings where it performs best. For example, use it to summarize or generate information where a human will be in the loop (code generation, summarizing documents, and providing support information). Don’t try and use it to detect adversarial inputs, and don’t blindly trust the output. Finally, don’t start replacing or ignoring “classical” machine learning approaches in favor of GenAI just because it’s new right now.
Based on the progress made over the year, what are your expectations on how the next year will look like? In your opinion, is the progress exponential or more steady?
Rakesh: It’s hard to predict whether it’s exponential or linear, and it’s likely that new hardware innovations will increase the performance of these models. I see exponential growth in the application of GenAI for a varying array of use cases over the next few years. We are still scratching the surface of how we can use this powerful piece of technological innovation.
I am anxiously waiting to see what the competition in this space is going to yield in the next few years. Major players are all doubling down on GenAI, and this competition is going to accelerate the performance of these models. It’s also possible that applications will start using multiple models behind the scenes to improve their efficacy.
I also expect to see privacy requirements around data, more control over inputs, and quick and effective mitigation of hallucinations to drive attempts to generate domain-centric localized LLM models constrained to an environment even though the inputs may be limiting and the overall effort cost-prohibitive.
Chaz: We’ve seen an explosion in interest and research in this space. On the security side, I believe we’ll continue to see new weaknesses in this technology that get exposed over the next several years. Fundamental flaws are already being explored (e.g., prompt injection), and continued research will likely find more challenges.
As with other new technologies, I think we’ll continue to see GenAI used in new and unexpected ways—some may be good, and others may not be so great. To facilitate this, more frameworks and SaaS offerings will pop up to make working with these technologies easier for groups that lack access to hefty computing resources. I think there will also be a lot of work to make GenAI technologies more efficient (like improving LLMs by training on less data, operating with less computing and memory).
I think the GenAI craze has been spurred on by the “magic” of ChatGPT. Asking it a question is very accessible, and the answers it generates seem really impressive—even when they’re wrong. Combined with the increasing ability to analyze or generate different types of inputs/outputs (e.g., images, PDFs, etc.), I think it’s been incredibly easy for folks to find new and interesting ways to use this technology. I’m excited to see more people interested in the AI/ML space.
Takeaways
A lot has happened with Generative AI over the year, and while we can’t always predict the future, a couple of ideas will likely drive progress during 2024:
- GenAI has changed a lot over the year so it’s likely going to keep changing at a rapid pace. Stay tuned!
- It’s gotten easier for more audiences to build on top of LLM. Cottage industries will likely explode in the near future.
- The technology still lacks semantic understanding. You still need to involve a human in every application. Most security applications have and will continue to focus more on tasks such as data summarization in non-adversarial environments.
For more insights into incorporating AI into a cybersecurity strategy, be sure to check out Devo’s Definitive Guide to AI and Automation Powered Detection and Response.