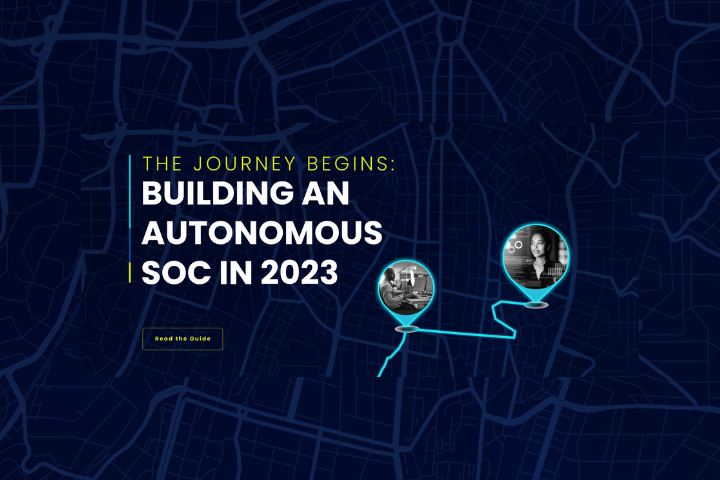
eBook Journey to the Autonomous SOC
Unlock the power of automation. Discover the key factors for automating threat detection, hunting, and response in this ebook.
Devo takes a customer-first approach: From pre-sales to renewal, we’re always on your team. Whether it’s gigabytes, terabytes, or petabytes, we have the process and expertise to migrate any organization to Devo.
Think there’s no way to simplify SIEM migration? Think again. Our experts make migration, onboarding, setup, and deployment a seamless experience. Devo employs a proven process custom-fit to your environment.
The Devo Learning Hub is our self-paced learning portal. Support your team and accelerate time to value with courses, content, and hands-on labs within a sandbox environment.
Empower your team with access to Devo Connect, our thriving online community. Ask questions, share best practices, implement new use cases, accelerate troubleshooting, and better protect your organization.
Devo’s privacy program is designed to be flexible and to respond to the changing landscape of global privacy laws and regulations.